Lunchtime talk write-up
Posted on Tue 12 Dec 2017
AI For Sceptics
Studio resident Tim Kindberg has been looking for a way of putting the actual capabilities of artificial intelligence (AI) – as opposed to the hype – into perspective.Tim has written a blog article about AI, The Scent of Data: https://matter2media.com/blog/scent-of-data/Five Things I Learned:1.
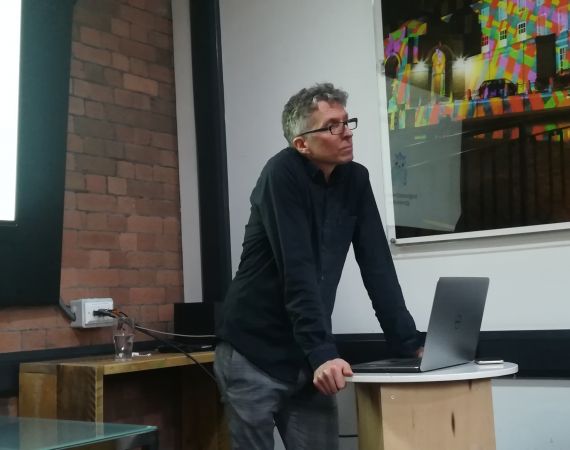
Posted by
Studio resident Tim Kindberg has been looking for a way of putting the actual capabilities of artificial intelligence (AI) – as opposed to the hype – into perspective.
Tim has written a blog article about AI, The Scent of Data: https://matter2media.com/blog/scent-of-data/
Five Things I Learned:
1. Tim uses the analogy ‘what AI has achieved through machine learning is not like human thought so much as a new type of smell – of data’. Tim adds ‘AI is much less conceptual and less cognitive than a dog’. Most ‘intelligent’ robots are simply following a set of rules. True artificial intelligence needs to be able to recognise and understand what it is looking at.
2. The complexity of human thought and communication can be demonstrated through the idiosyncratic way humans interpret and process symbols, from Shakespeare’s use of metaphor and language, to William Blake’s painting Newton, which depicts a male figure hunched over a scroll and compass, arguably turning his back on nature for maths and physics. Is AI capable of understanding the symbolism and meaning behind such complex language and imagery?
3. There have been a number of ‘AI winters’ where funding and interest had dried up, but at the beginning of the century as a result of big data and increased processing power, there is now a Tsunami of AI development based on machine learning and vast computing power. Deep learning itself has been around since the 1960s. Google have invested millions into AI for health care, but Tim isn’t convinced it’ll replace doctors anytime soon.
4. Machine learning is however, particularly efficient at recognising handwriting, speech, surveillance and marketing. A big caveat is that a huge amount of human input needs to happen hundreds of times before it can work and even after all that laborious input, deep neural networks are easily fooled. Tim showed us an example, where a computer is asked to identify a baseball, but often mistakes it for a coffee cup depending on the lighting and angle of the photograph.
5. There are also wider political and ethical implications of using AI. Programming unconscious biases into AI machine learning is a huge issue; Google’s face recognition system came under fire when it was discovered that it was incorrectly labelling photos of certain ethnicity groups as gorillas. These biased bots aren’t very appealing. Tim also questions where all this input data comes from and whether people are aware that their data is being used in this way.